Telemedicine and artificial intelligence related to vision screening
Current state and future prospects for German optometry
Purpose: This paper aims to evaluate whether telemedical models or examination and prevention models based on artificial intelligence offer German optometrists a chance to increase their status as vision care providers and to provide better eye care in Germany.
Material and Methods:
Both a systematic literature review and an expert survey were conducted. Study results of deep learning vision screening applications in the fields of glaucoma, age-related macular degeneration, and diabetic retinopathy as well results of expert interviews are discussed.
Results: The study results and expert interviews demonstrate that cooperation between optometrists and ophthalmologists in a telemedical network is an essential tool for improving the eye health care of the population and relieves ophthalmologists of routine tasks. The first telemedical cooperative program between German optometrists and ophthalmologists demonstrates a successful cooperation.
Conclusion: Artificial intelligence (AI) is increasingly finding its way into the field of vision screening. Telemedicine and the electronic patient medical record play a unique role. They enable German optometrists to secure and expand high-quality service offerings in the field of optometry.
Introduction
Against the background of the worldwide increase of sight-threatening eye diseases the question of optimal health care arises. This is due in part to the changing demography and aging in industrial societies, and the rapidly changing geographical supply structure (urban-rural flight). At the same time, there is a rapid evolution of technical solutions, including improved accuracy of diagnostic tools. For some AI procedures, the evaluation of their results by artificial intelligence achieves a diagnostic accuracy that exceeds that of ophthalmic professionals.
What role can technology in the form of telemedicine and artificial intelligence play in improving eye care and vision screening by optometrists in Germany? This work is intended to show the most important technical systems currently available, with a special focus on the systems already available to German optometrists. These systems could not only increase the contribution to preventive care in the field of ocular health, but they also offer a further interface in the practical education and training system for optometrists. Telemedicine could offer a great opportunity to achieve stronger cooperation between optometrists and ophthalmologists through these interfaces, for the benefit of patients, because structurally weak regions in Germany already have a shortage of doctors and require long distances to reach a doctor´s office.1 At the same time, it could make a significant contribution to the further development of the clinical qualification of German optometrists.
Material and methods
The paper uses two different approaches to determine the scientific state of the art: a search in scientific databases and qualitative expert interviews of market providers for telehealth services for vision screening in Germany and of market providers using artificial intelligence to support optometrists.
Vision screening by optometrists in Germany
In Germany, optometrists are part of the health sector. There are no legal restrictions on the use of any type of examination equipment. Not allowed is the use of diagnostic and therapeutic drugs. Optometrists in Germany are allowed to make a tentative diagnosis in cases of abnormalities of the eye.2 Optometrists in Germany perform screening procedures to detect abnormalities of the eye as well as comprehensive eye examinations. The clinical guidelines of the professional body for ophthalmic optics and optometry in Germany explain the differences between screening procedures and a comprehensive optometric eye examination.3 Nevertheless, the non-uniform training of opticians and optometrists in Germany reduces the opportunities for greater involvement in the field of eye-specific health care.
Internationalization of higher educationin Germany
The interlinking of clinical optometric content in the bachelor‘s and master‘s degree programs does correspond to the international job description of the „primary eye care provider“. However, clinical optometric practice under supervision with a final independent examination by the professional association, which is common in international university education, is not yet established in Germany.4
Telemedicine
Telemedicine is the application of communication and information technologies in the health care system between participants in different locations.3 Synonyms for the term telemedicine are e-health, and health telematics. Special features of the new digital technologies result from this spatial separation, but also from the possibility to vary the time component. The contact can take place simultaneously (real-time) or delayed by hours or days (store and forward). Applications of telemedicine are aimed at medical care, i.e. diagnostic and therapeutic support across spatial distances.4 The most important forms of telemedicine applications are telediagnostics, telecooperation, teleconsultation, teletherapy, telemonitoring, teleconference, and telemedical emergency care, as shown in Figure 1.
Telemedicine in Germany
The development in Germany is characterized by strict laws and regulations of the medical professional associations. Despite numerous legislative initiatives, such as the implementation of an electronic health card, the self-governing bodies of the German healthcare system are very slow. It is therefore not surprising that in 2018 Germany ranked second to last in an international comparison in a study by the Bertelsmann Foundation, which analyzed and compared the status of digitization in the healthcare sector of 17 countries in the areas of policy readiness, digital health readiness and actual data use.7The reasons for the poor performance of the German health care system can be found in the strongly corporatist and federalist German health care system.8 Despite numerous individual telemedical projects funded by the German government, these do not find their way into health care.
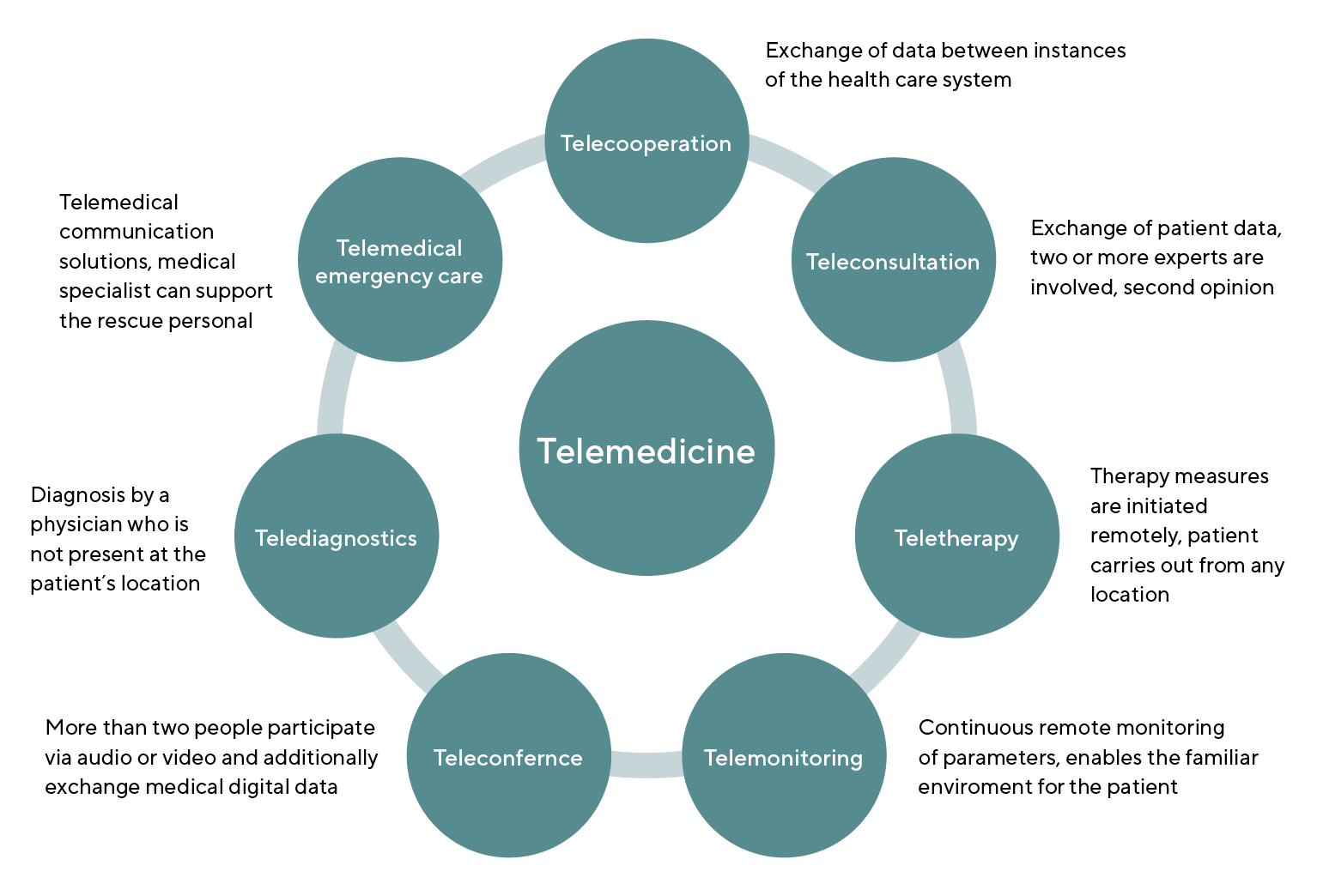
Teleophthalmology and effects of the COVID-19 pandemic
In the field of ophthalmology it is reported that during the current COVID-19 pandemic the demand for teleophthalmic solutions has increased dramatically.9 The establishment of teleconsultation platforms minimizes the risk of infection for all outpatient medical specialties, including ophthalmological practices and clinics, through close contact at examination units, in waiting areas, or on the way to examination sites.
Artificial intelligence (AI)
Artificial intelligence refers to the ability of a machine to perform cognitive tasks that we associate with the human brain.10 AI has many subtypes, as shown in Figure 2. However, only with the enormous technical development of the four factors of Big Data, Storage and Computer power, and Global Networking on the Internet of Things, could the branch of deep learning develop.11Deep learning is also a method of statistical learning; the term deep in this context refers to the concept of data through layered representations. Thus, it is not about an attainable understanding, but about a multitude of successive representation layers. Today, these representation layers can comprise hundreds of successive representation layers.12 The model after which the learning of the representation layer takes place is called a neural network. These neural networks consist of multilayered layers. Chollet emphasizes that deep learning models are not replicas of the brain, but that deep learning is a mathematical framework for learning the representations using data.12 Since the class of convolutional neural networks (CNN) within deep learning proved to be particularly useful for image recognition, these methods achieved enormous progress in medical fields that are strongly image-oriented, such as ophthalmology, dermatology, and radiology. In the field of ophthalmology, artificial intelligence based on deep learning is applied to fundus images, optical coherence tomography, and visual fields. The main objective is to screen and diagnose the most important eye diseases such as diabetic retinopathy, glaucoma, and age-related macular degeneration. Many studies prove the efficacy.
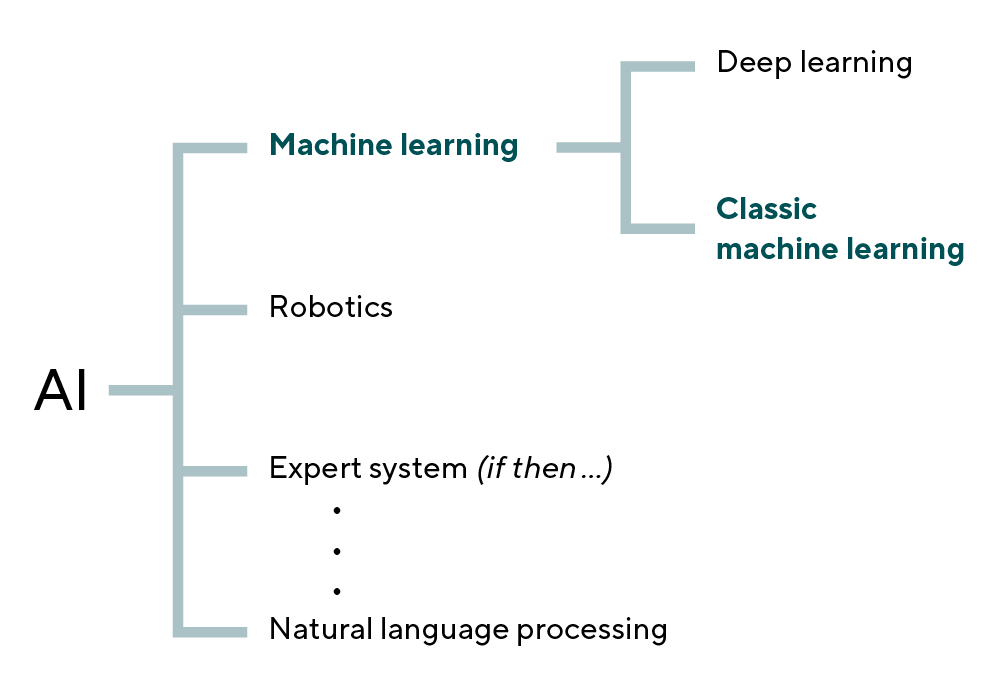
Deep learning and the examination of the optic nerve head in glaucoma
Generally, glaucoma tests can be divided into those that detect structural or functional glaucomatous changes.13 Tan et al. believe that the challenge is that screening does not examine and evaluate these changes over time, but only at a specific time. Despite this background, they consider the use of photography and optical coherence tomography (OCT) as a promising strategy for structural examination. Digital color fundus images have been widely used in recent years to support the diagnosis of glaucoma, as they are broadly available and are much less expensive than optical coherence tomography.14 Fundus photography supports the examination of the optic disk and nerve fiber layer, cup/disc ratio, bleeding and notches at the edge of the optic disk, and at the same time serves as reliable documentation and exact comparison in subsequent examinations. The Glaucomatous Optic Neuropathy Evaluation Project has already shown that subjective evaluations of monocular images of the optic nerve head offer equally high diagnostic accuracy in glaucoma compared to stereoscopic images.15 Li et al. evaluated a DL algorithm for the detection of referable glaucomatous optic neuropathy (GON) based on fundus photography.16 They showed that a deep learning algorithm can detect GON with high sensitivity and specificity, 95.6 % and 92 % respectively, and with an area below the operative receptor characteristic curve (AUC) of 0.986.16 In a study by Gonzalez-Hernandez et al. the technique of deep learning is applied to automatically determine the position and shape of the optic disc and used for edge detection to optimize the reproducibility of the Laguna ONhE method.17
The program, which is operated by the Danish company Retinalyze on a web-based platform and offered in Germany by a Swiss licensing company as a screening tool as part of its fundus image analysis, is based on the following underlying concept: The program uses fundus images to determine the hemoglobin level of the optic disc. The color differences are a measure of tissue thickness. The greater the thickness of the tissue or its concentration of hemoglobin, the more intense the color, and the thinner the tissue or the less advance of blood, the whiter it will appear.18 The blood vessels of the neuronal tissue serve as a reference measure of color. By comparing the color of the neuronal tissue with these blood vessels, the amount of hemoglobin in each region of the tissue is estimated in order to create a representative map of the local distribution of hemoglobin from the different ratios.13 The vertical cup/disc ratio is estimated from the topographic distributions of hemoglobin and a so-called glaucoma discriminant function (GDF) is used to calculate a risk score for glaucoma. Difficulties are posed by cases of peripapillary atrophy and peripapillary myopic atrophy as in these cases the edges of the optic disk have to be demarcated by hand. A further limitation is when the retinal nerve fibers in the area of the lamina cribrosa are myelinated.18 Despite the exact analysis of individual parameters by artificial intelligence, Akkara et al. believe that a comprehensive AI for glaucoma should evaluate all parameters including IOP, optic disc, angle of the anterior chamber of the eye, and visual field. They further state that such an AI system does not yet exist.19 Diener also noted that currently no single examination procedure alone has sufficiently valid data to diagnose glaucoma with certainty.20
Deep learning and age-related macular degeneration (ARMD) and diabetic retinopathy (DR)
The earliest phase of ARMD is typically observed as the presence of (asymptomatic) macular drusen, often found incidentally during fundus examinations or fundus imaging.21 Drusen are extracellular deposits located at the junction of the RPE and Bruch‘s membrane.22 Clinically, hard and soft drusen are distinguished; those that are white or yellowish hard drusen are well defined, and the presence of small drusen is not in itself a diagnosis of ARMD as they are common in normal aging. Drusen are rarely homogeneous in their composition. Due to their yellow color and brightness on color fundus photographs, drusen are distinguishable to the human eye, but computer algorithms for automatic detection must be robust to the presence of other pathologies that appear similarly bright as hard exudates.22 Fundus-based DL algorithms based on the Age-Related Eye Disease study (AREDS) data set were developed by Burlina and Grassmann. The algorithm developed by Burlina et al. for automatic detection and assessment of ARMD in referable ARMD showed results comparable to the performance level of human experts.23 A similar deep learning System was built, which also used the AREDS data set to develop its system and used the Augsburg data set for testing, which consists of 5555 fundus images collected in the Augsburg region as part of the collaborative health research. This algorithm also shows a high classification accuracy in the AREDS data that surpasses human graders.24 However, Ting et al. point out in the context of this study that the AREDS data set consists mainly of people older than 55 years and therefore many dominant macular reflexes were misinterpreted as neovascular ARMD in the tested foreign data set, which underlines the importance of training DL algorithms with different clinical data sets and a wide range of phenotypes and patients.25 Grassmann et al. also do not suggest in their study that this classification system should be used by an ophthalmic clinic or practice.19 In contrast to diabetic retinopathy, where the majority of studies focus on fundus photography, artificial intelligence in ARMD also focuses heavily on OCT.25,26 For Germanoptometrists, optical coherence tomographs are certainly not standard equipment at present.
RetCAD v.1.3.0 (Thirona, Nijmegen, Netherlands) is an example of a deep learning system for automatic detection of ARMD and DR. It is CE-certified and commercially available. The system can be used on a telemedical platform offered in Germany. The AI algorithm based on the technique of a convolutional neural network analyzes color fundus photographs for the presence of age-related macular degeneration and diabetic retinopathy. As output values, the software gives a value for the image quality and a score for the presence of ARMD and DR. The score indicates the probability that the patient should be referred to an ophthalmologist. So-called „heat maps“ indicate the location of the detected image anomalies and thus serve as a visual aid to control critical areas on the retina. The point values range from 0 to 100 and are based on the AREDS classification protocol for ARMD and the ICDR classification protocol for DR. A point value within 30-50 is given as the threshold zone for the decision of referral or non-referral. Low selected thresholds achieve high sensitivity with the disadvantage of more false positive referrals, higher thresholds lead to a high overall specificity at the expense of false negative referrals. A study by González-Gonzalo et al. concludes that the system performs comparably to human experts when DR and ARMD are detected simultaneously.27
Telemedicine and AI applications for optometrists in Germany
Through the evaluation of German professional journals in the field of ophthalmic optics and optometry for the years 01/2018-06/2021 and the analysis of exhibitors at the German Opti 2020 trade fair in Munich the current commercial providers of telemedical services on the German market summarized in Table 1 could be identified. All providers of telemedical devices used artificial intelligence. However, the providers work simultaneously either with local ophthalmologists or with their own so-called „backup“ ophthalmologists in the background. If a finding is not known to the artificial intelligence, image reading by the involved ophthalmologist can take place. This means that the findings optometrists receive from the networks are backed up by specialists. This is because the German optometrist, when discussing a finding with the patient about any abnormalities, must explain to the patient that he or she will only receive a final diagnosis from the ophthalmologist. It is not necessary for the providers to specify the type and scope of the AI used.
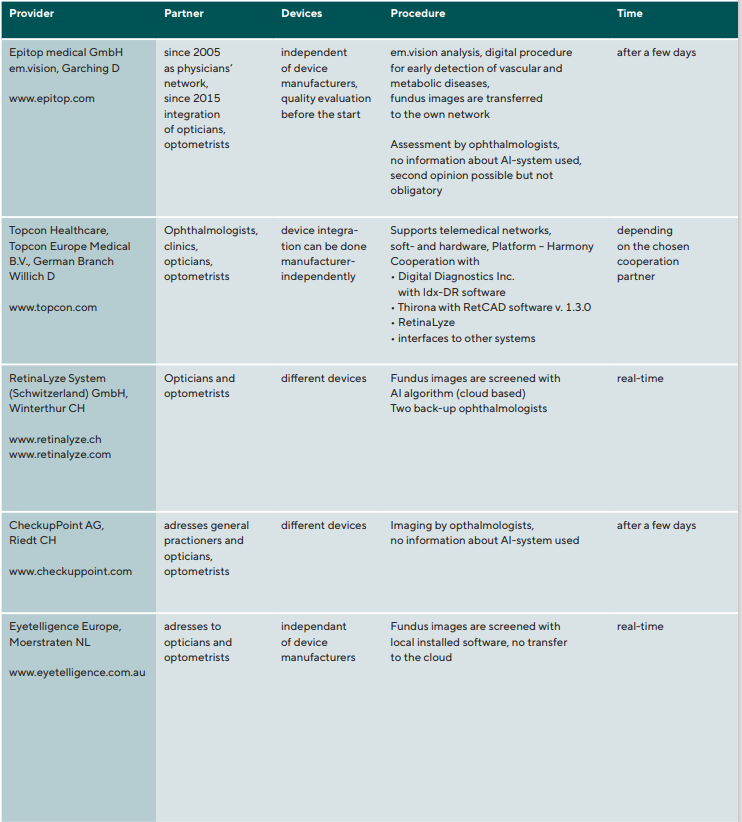
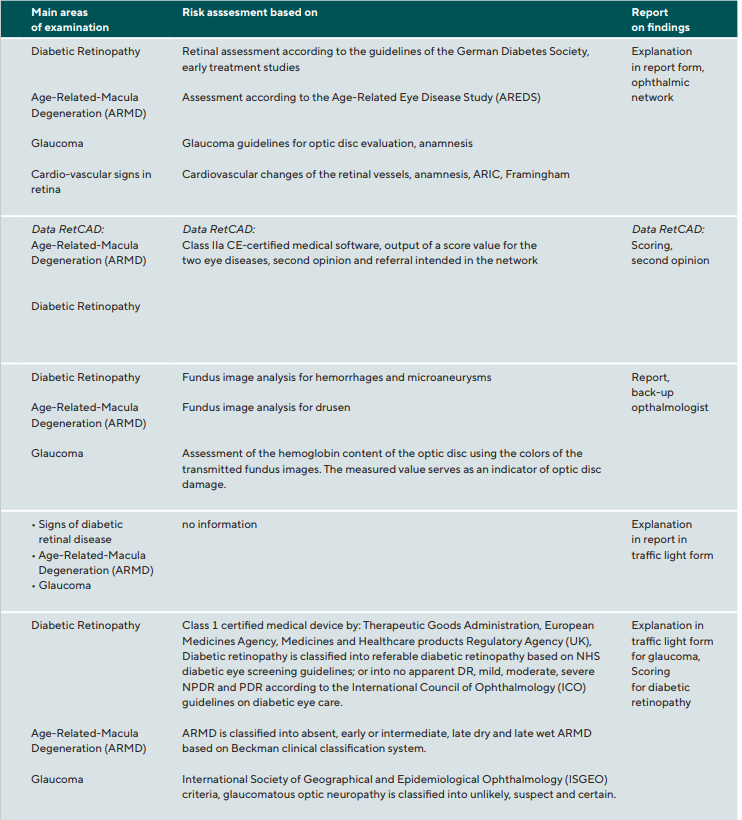
Epitop Ltd
One of the earliest telemedical programs in Germany was developed by the company Epitop in Garching, which has expanded its network for opticians and optometrists based on a medical network and a self-developed patient file in 2015. The images acquired by the opticians or optometrists using a fundus camera or laser scanner are captured, transmitted and evaluated by the ophthalmologist connected in the network. The type and scope of the AI software used is not published by Epitop. A number of device manufacturers integrated in the network keep the technical barrier as low as possible. Usually, the optometrist discusses the evaluation with his patient when it is transmitted via telemedicine after a few days. A recommendation for any further intervention is included in the report. The advantage of this pathway lies in the integration of the optometrist into the ophthalmologic network, which is oriented to the geographical location of the partners in the network if possible.The primary conditions included in this pathway are diabetic retinopathy, ARMD, glaucoma, and also changes in the retinal vessels, which may indicate cardiovascular diseases of the patient. A regional reference can be offered to the customer if necessary and desired by integrating the optometrist into the network.
Topcon Germany Medical GmbH
A solution for digital networking of ophthalmologists and optometrists which is based on manufacturer-independent software and at the same time supports the integration of artificial intelligence has been realized by Topcon Deutschland Medical GmbH. The platform for telemedical services, marketed under the name Harmony, makes it possible to link the most diverse diagnostic devices of an ophthalmologist’s or optometrist‘s practice locally or at different locations. At the same time, the cloud-based software allows the application of artificial intelligence and offers interfaces to these providers. The collaboration with IDx, a private AI diagnostics company in the United States, enables the use of their AI system IDx-DR to detect diabetic retinopathy in digital fundus images. IDx-DR is the first AI-based diagnostic system with FDA approval in the U.S. and a Class IIa CE mark for autonomous detection of diabetic retinopathy. It is also possible to integrate the AI software RetCAD into the system. In Germany, one can already refer to a successfully implemented health care network between ophthalmologic practices and an optometrist. The measuring and testing devices of an eye center managed by an optometrist are linked via the digital platform to the ophthalmic measuring devices of two ophthalmic practices as well as other affiliated optical practices. The local networking relieves the ophthalmologists in the field of preventive medical checkups and screenings and offers the patients fast access to specialist medical care if necessary.
Retinalyze System A/S
The Swiss company RetinaLyze is the licensee of an AI software from the Danish company Retinalyze System A/S, based in Hellerup, which focuses on the evaluation of fundus images using artificial intelligence. The optometrist uploads their fundus images to a web-based portal and receives a written assessment in real-time, which he can immediately discuss with the patient. If there are unattributable results from the AI, the back-up ophthalmologist is called in and contacts the optometrist, which offers additional certainty of assessment in cases of doubt. Two ophthalmologists work for all system partners in Germany and no regional or local networking is integrated within the system. If there is a „referable“ assessment, the patients must make the appointment with the local ophthalmologist themselves if the optometrists do not have access to their own network of ophthalmologists or clinics. The AI software used is mainly applied to check diabetic retinopathy, age-related macular degeneration, and glaucoma.
Checkuppoint AG
Another provider of a telemedical service is Checkuppoint AG, a company based in Switzerland. According to the documentation, the diagnosis is made by ophthalmologists and the assessment in the form of a report by means of traffic light marking on the optometrist. The focus of the findings is on clinical signs of diabetic retinal diseases and ARMD. A disadvantage appears to be that the optometrists may have to rely on their own reference network to ophthalmologists or clinics. No information is given on the type and scope of the AI software used.
Eyetelligence
Since mid 2021, the Australian company Eyetelligence offers its workplace software eyetelligence-Assure in Germany. The software is certified by various agencies (Table 1) as a class 1 medical device. The manufacturer, whose software is installed on the local computer of the ophthalmologist or optometrist and thus does not store data in the cloud, requires fundus images for screening. The most common fundus cameras of the major manufacturers (DRS, Nidek, Topcon, Zeiss) are supported. The software screens the fundus images for glaucoma, ARMD, and DR. For glaucoma detection a traffic light system classifies the risk level and for DR classification a rating scale is used. Further data, such as the accuracy of the screening program for the individual eye diseases and the support of the users in case of non-detected abnormalities, are not available at the present time (July 2021).
Results of the expert interviews
The expert interviews were conducted by telephone in June and July 2020.The following four main topics were defined for the interview guide: (1) use of telemedical support and artificial intelligence, (2) advantage and disadvantage of telemedical support in optometric screening in reference to parties involved, (3) implementation of telemedical models in companies, and (4) deepening and broadening competences.
As a focus, this paper presents the most important expert recommendations and specific experiences from initial practical implementations and scope of further education and training opportunities. If a company is planning to enter a telemedical network (TN), the expert interviews have shown that there are challenges but also clear opportunities for the company. The interview partners see the challenges of implementation in a clearly defined measure catalog, the step-by-step implementation of which is aligned with the future strategy of the company. The recommendations for the implementation process point to a clear definition of goals and milestones at the beginning of the process. The basis is an analysis of the own organization, the existing resources in the company from the spatial to the personnel and financial equipment, as well as the development and training of the technical expertise in the company. This professional expertise includes optometric expertise as well as communication skills towards customers and ophthalmologists. Finally, the optometrist must gain an overview of the technical equipment units used and their advantages and disadvantages. If necessary, attention must be paid to the existing interfaces to the various network providers; certain devices can often be integrated in a particularly user-friendly way, whereas, for example, when transmitting image documentation, these have to be uploaded separately onto the network, which can considerably impede the workflow of the company.
Overall, the experts consider the right approach to implementation to be essential for success and sustainability. They generally consider artificial intelligence to be very relevant and this statement is confirmed by the fact that all companies offering telemedical services for optometrists in Germany already use AI. For the optometrist using a telemedical network, additional professional security is provided in Germany since the considered telemedical networks, due to the legal situation, either generally provide for observation by an ophthalmologist or, in case of an unclear classification by the AI, the so-called backup ophthalmologist is included. This form of telemedicine network design offers a great opportunity for better integration of optometrists into preventive care especially when telemedicine networking enables local networking of ophthalmologists and optometrists and the joining of the two parties is not based on competition but on the partners‘ recognition that they are working together for the prevention of ocular health and the well-being of patients and clients. One interview partner (CEO of a TN) states that the networked ophthalmologists have recognized that the detection of an abnormality is only ten percent of the patient‘s history, but that the ophthalmologists are then required to treat the patient.
Special attention must be paid to internal and external marketing. Marketing communication begins within the own company towards the employees. Here workshops and training courses can be offered so that the potentials and advantages can be communicated clearly to the customers. This also includes the clear delineation of one‘s own fields of competence with regard to the assessment of the observed structures and their classification (conspicuous or not conspicuous). On the other hand, patients do not yet have extensive knowledge of the scope of activities of optometrists and, especially in the case of AI, do not yet have complete confidence in this area of application. Concerns about data security must also be considered as the telemedical processing of personal health data requires special care and technical security measures. The health data may only be communicated or transferred with the consent of the person concerned and is particularly protected by the General Data Protection Regulation (GDPR).
The experts urgently recommend coordination with the local cooperating ophthalmologists. This will allow the definition of a standard for the description and documentation of the abnormalities and will facilitate future communication with the ophthalmologists. There is no doubt that the practical implementation of screenings by optometrists is seen as the most important factor to expand the optometrists’ professional knowledge. However, the optometrists should perceive participation within a telemedicine network as a fundamental opportunity to compare their own knowledge from the reports submitted back to them with each transferred case and to further develop and build on this knowledge. It was criticized that many of the training measures offered were not as well perceived as the providers would like. The reasons are seen in the scarcity of resources, time, and financial expenditure. Against the background of the COVID-19 pandemic, some companies are already implementing a change towards more extensive online offers through webinars and accessible podcasts.
Conclusion
It is recommended for German optometrists to fundamentally deal with the possibilities of telemedical networks and the integration of artificial intelligence. Education and training out of these networks are great opportunities for continuing professional development. There are many advantages for patients, optometrists and ophthalmologists. Before the optometrist enters such a network, he should have a plan, a concept and a strategy.
Acknowledgements
I would like to thank Prof. Dr. Anna Nagl from Aalen University, Germany, and Prof. Dr. Tony Cavallerano from New England College of Optometry in Boston, USA, for their helpful suggestions and constructive comments. Very special thanks are due to all who participated in my interviews.
659-667.